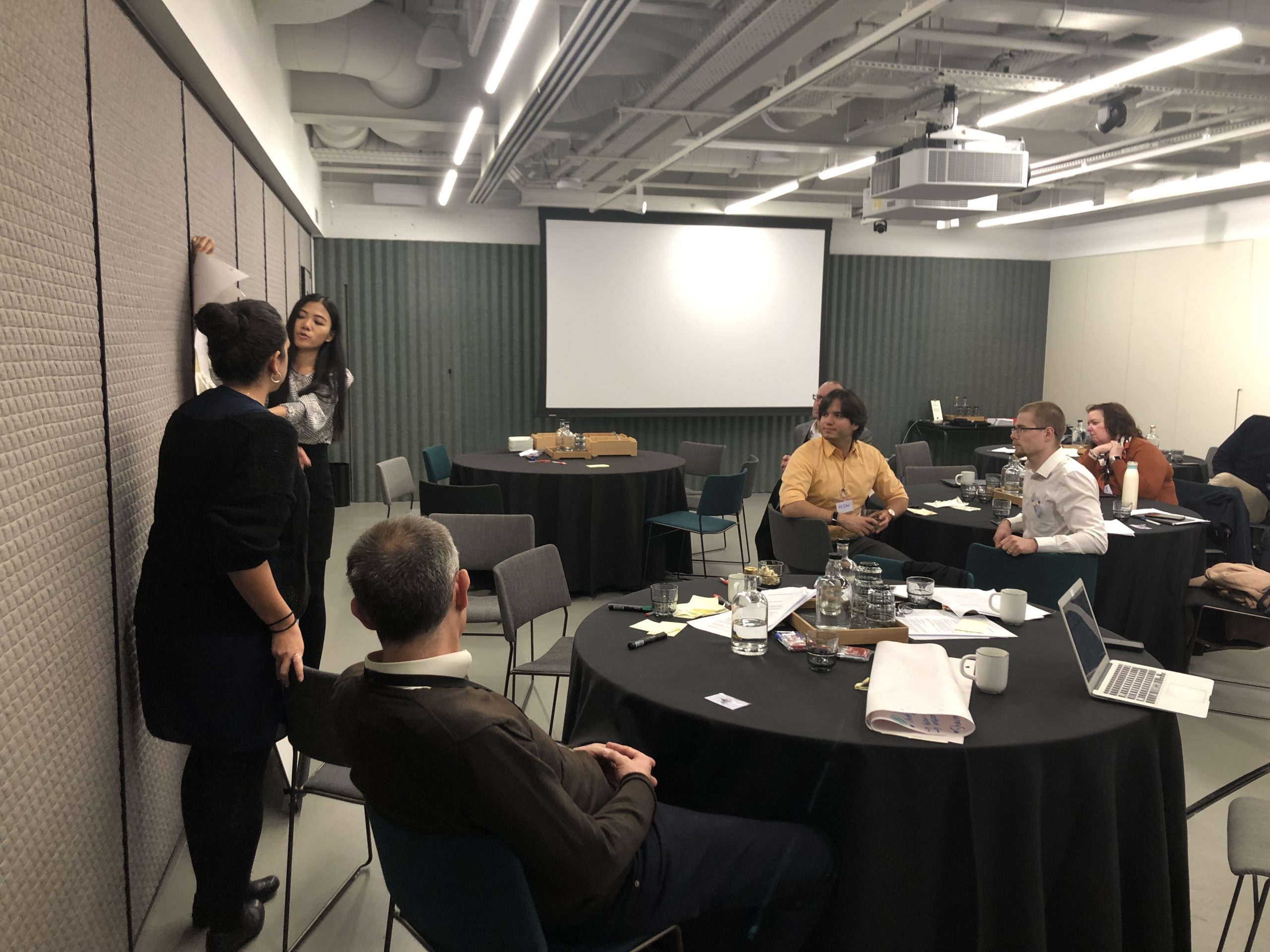
Public AI Procurement Guideline Workshop with Office for AI
Governments are increasingly seeking to capture the opportunities offered by AI to improve public-sector productivity and the provision of services to the public and to stimulate the economy. AI holds the potential to vastly improve government operations and meet the needs of citizens in new ways, ranging from traffic management to healthcare delivery to processing tax forms. However, governments often lack experience in acquiring modern AI solutions and many public institutions are cautious about harnessing this powerful technology.
https://www.weforum.org/whitepapers/ai-government-procurement-guidelines
World Economic Forum (WEF) created the Guidelines for AI procurement to help governmental institutions in AI procurement, and the UK will be one of the first countries to test these guidelines. With the Draft Guidelines for AI Procurement, the Office for Artificial Intelligence in the UK held a workshop in London at 16th October 2019 to gather feedback and improvement suggestions on these guidelines.
As a tech supplier for the UK government who won and completed a GovTech Challenge project, Qualimental Technologies was invited to the workshop to give feedback on these guidelines.
Draft Guidelines:
- Explore procurement processes that focus on the challenge rather than a specific solution
- Define the public benefit of using AI while assessing risks
- Include your procurement within a strategy for AI adoption
- Incorporate references to legislation and codes of practice in the invitation to tender
- Articulate the technical feasibility and governance considerations of obtaining relevant data
- Develop a strategy to address technical and ethical limitations of using training data
- Conduct procurement with diverse multidisciplinary teams
- Focus on mechanisms of accountability and transparency throughout the procurement
- Consider the life-cycle management of the AI system
- Creative a level and fair playing field for suppliers
As a tech supplier who is experienced in artificial intelligence development, Qualimental Technologies presented the life-cycle of an AI project and the important factors that the procurement officials should consider at each specific point in the life cycle.
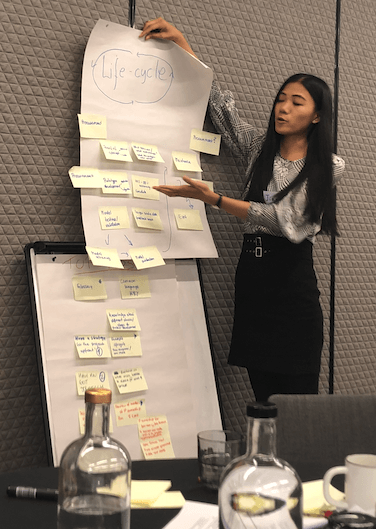
The life-cycle of a typical AI project
Idea/Requirement
Any project starts with a problem that needs AI to solve or a need that AI could satisfy, for example, use AI for detection, automation and prediction.
Proof of concept
An AI project comes with a set of technical hypotheses which needs to be validated before developing a product. And this is commonly called proof of concept or feasibility assessment. In addition, it is also advised to involve a user-centric design of the end solution.
Prototype Development
After validating the hypotheses using preliminary developed models and algorithms, the next phase is to continuously develop and optimise models and algorithms and develop a prototype.
Model Testing/Validation
After the prototype is developed, the models need further testing and validation, generally after adding additional training data (either generated from users or externally).
Model Refinement
Depending on the results from the model validation, it might need to be refined before deploying it into production environment.
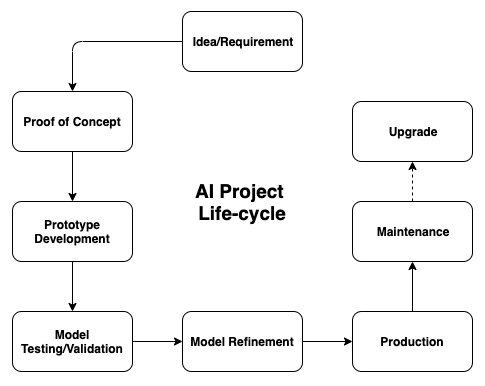
Production
When the model accuracy reaches the desired level (the exact percentage depends on the specific use case, but we consider 95% to be a threshold for reliable production use, while sometimes lower accuracies around 85% can also be acceptable). In order to use a model in production, it is normally wrapped into a web application or API, which exposes an API endpoint, to return the processed results.
Maintenance
Same as any other application, the API wrapper, as well as AI models, also need maintenance. The work mostly includes data engineer checking logs and debugging or data scientist verifying the results from the model.
Upgrade
The purpose of the maintenance is to guarantee reliable performance and make sure that the service is delivered as expected, while the purpose of the upgrade is to add additional functions/features. If the additional functions/features can be supported by the original AI model, then it only requires application development work, but if the additional functions/features exceed the range from the original AI model, then it needs to start from a proof of concept.
Leave a Comment